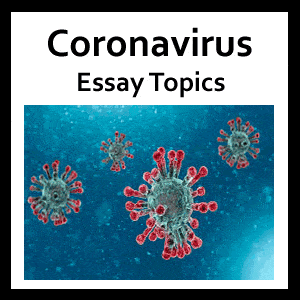
Using the spike protein feature to predict infection risk and monitor the evolutionary dynamic of coronavirus. To see Coronavirus Essay Topics go to the https://smartcustomessays.com/coronavirus-covid-19-essay-topics-and-ideas/ website.
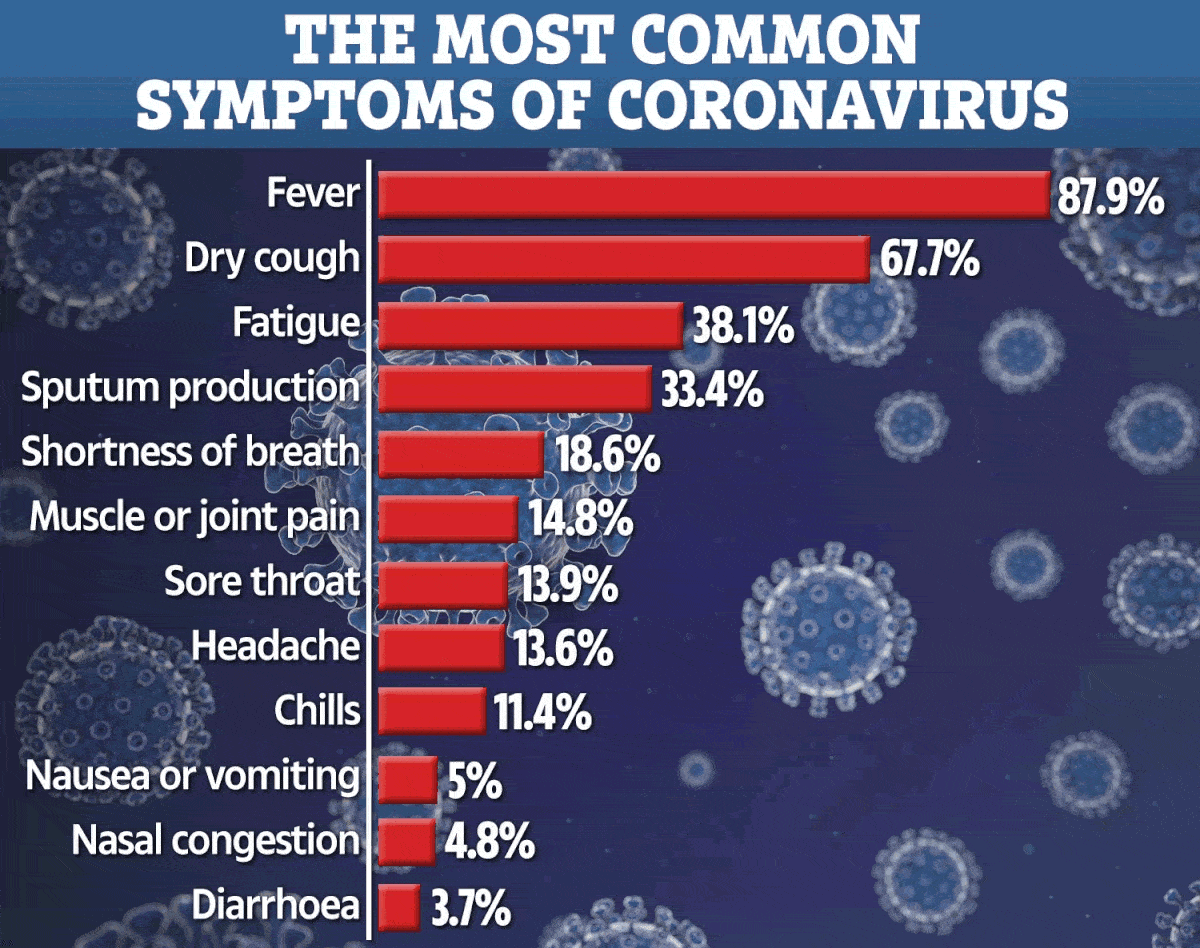
Abstract
Background
Coronavirus can cross the species barrier and infect humans which has a severe respiratory syndrome. SARS-CoV-2 with potential origin of bat is still circulating in China. In this research, a prediction model is proposed to evaluate the infection probability of non-human-origin coronavirus for early warning.
Methods
The spike protein sequences of 2666 coronaviruses were collected from 2019 Novel Coronavirus Resource (2019nCoVR) Database of China National Genomics Data Center on Jan 29, 2020. A total of 507 human-origin viruses were regarded as positive samples, whereas 2159 non-human-origin viruses were viewed as negative. To capture the important thing information in the spike protein, three feature encoding algorithms (amino acid composition, AAC; parallel correlation-based pseudo-amino-acid composition, PC-PseAAC and G-gap dipeptide composition, GGAP) were used to train 41 random forest models. The optimal feature with all the best performance was identified with the multidimensional scaling method, which has been employed to explore the pattern of human coronavirus.
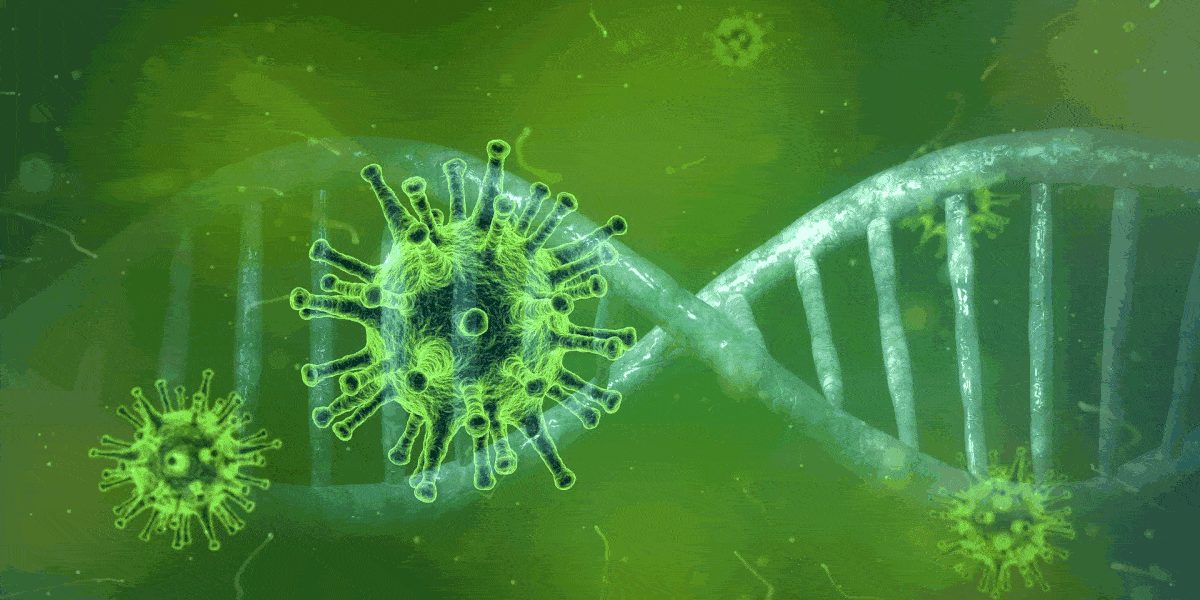
Results
The 10-fold cross-validation results established that well performance was achieved with all the use with the GGAP (g = 3) feature. The predictive model achieved the utmost ACC of 98.18% coupled with the Matthews correlation coefficient (MCC) of 0.9638. Seven clusters for human coronaviruses (229E, NL63, OC43, HKU1, MERS-CoV, SARS-CoV, and SARS-CoV-2) were found. The cluster for SARS-CoV-2 was very close to that for SARS-CoV, which implies that both viruses have the identical human receptor (angiotensin converting enzyme II). The big gap within the distance curve shows that the cause of SARS-CoV-2 just isn't clear and further surveillance inside field must be made continuously. The smooth distance curve for SARS-CoV shows that its close relatives remain in nature and public health is challenged as usual.
Conclusions
The optimal feature (GGAP, g = 3) performed well when it comes to predicting infection risk and could be accustomed to explore the evolutionary dynamic inside a simple, fast and large-scale manner. The study is advisable for your surveillance of the genome mutation of coronavirus inside the field.
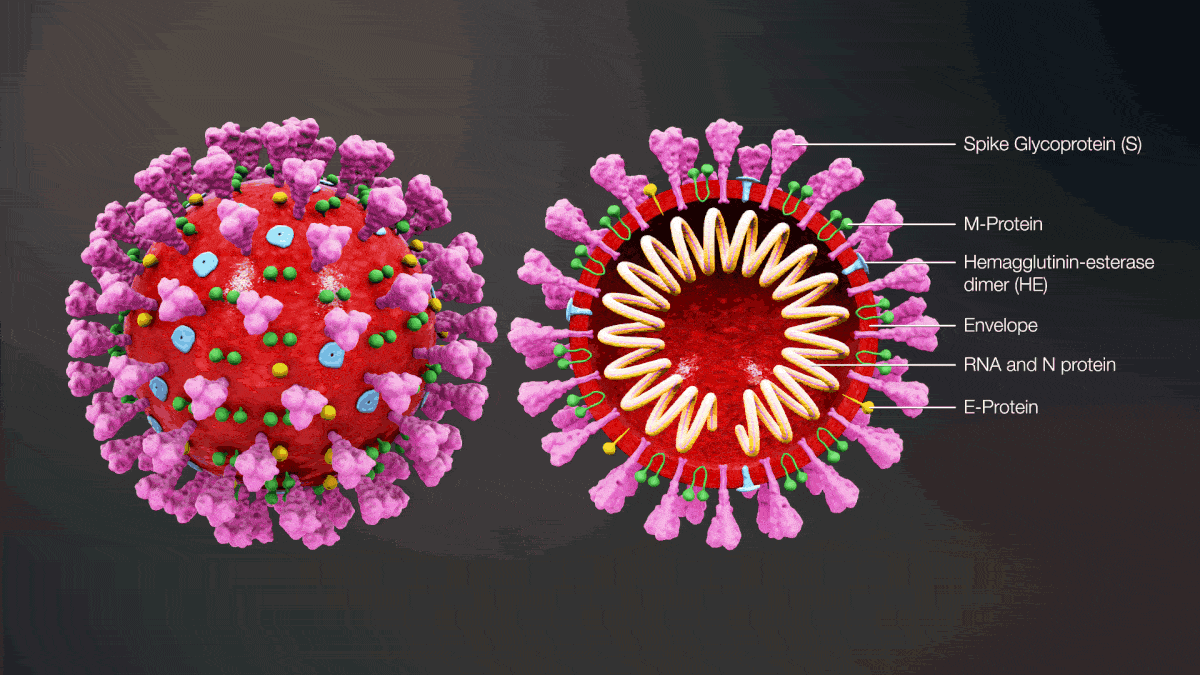
Background
Coronavirus Essay Topics (CoV) is probably the order Nidovirales and can infect humans, mammals, and birds [1]. The viral genome is composed of an optimistic stranded RNA, and its particular structures vary. The family Coronavirinae is divided into four genera: α, β, γ, and δ [2]. There are seven human coronaviruses: 229E (α-CoV), NL63 (α-CoV), OC43 (β-CoV), HKU1 (β-CoV), MERS-CoV (β-CoV), SARS-CoV (β-CoV), and SARS-CoV-2 (β-CoV). MERS-CoV, SARS-CoV and SARS-CoV-2 can infect humans and induce serious pneumonia with many fatal cases [3]. SARS-CoVs induced an epidemic in the world, and 774 fatal cases were reported [3]. Now, SARS-CoV-2 remains to be circulating in China [4,5,6].
As considerable coronaviruses happen to be isolated from bats as well as other animals, it really is belief that you will find there's viral gene reservoir in wild animals [7]. Coronavirus can directly cross the species barrier and infect humans rich in fatality [8]. As the antigen is novel for the human host, public health will be seriously challenged. The infection chance of coronavirus in animals needs to be analyzed along with a prediction model ought to be constructed for early warning. For this purpose, machine-learning methods appear to be ideal tools [9, 10]. The spike protein at first glance of the viral particle plays key roles inside binding with the cell receptor and membrane fusion [3, 11], where the host range is firmly determined [8]. In this research, we screened the options from the spike protein using three encoding algorithms and predicted the cross-species infection of coronaviruses with all the random forest method. Moreover, the suitable feature (G-gap dipeptide composition, GGAP, g = 3) was employed to explore the dynamic of evolution inside a simple, fast and massive manner.
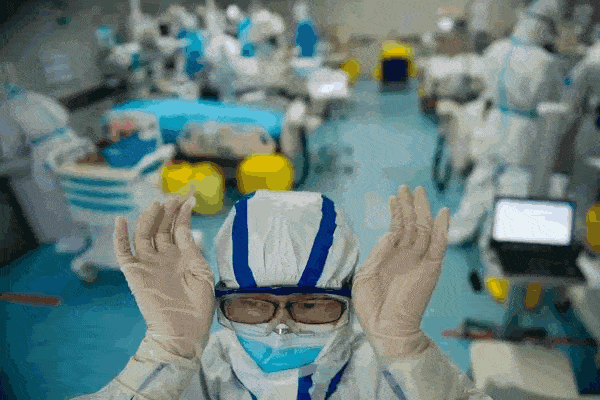
Methods
Dataset
The protein sequences of 2666 coronaviruses were collected from 2019 Novel Coronavirus Resource (2019nCoVR) Database of China National Genomics Data Center (NGDC, https://bigd.big.ac.cn/ncov) on Jan 29, 2020 [12]. These strains had complete genomes and were isolated between 1941 and 2020, and included SARS-CoV-2 strains. The information associated with these strains was summarized in Additional file 1. The 507 human-origin coronaviruses were regarded as positive samples, whereas the 2159 non-human-origin coronaviruses were viewed as negative.
Feature encoding algorithms
To capture the important thing information with the spike protein, we used three encoding algorithms from multiple perspectives, that is compositional information, position-related information and physicochemical properties (Table 1). The optimal feature using the best performance was shown from the multidimensional scaling method in R (MDS, https://cran.r-project.org/web/packages/MASS/index.html). The details with the feature encoding algorithms accustomed to encode the spike protein into feature vectors are listed below.
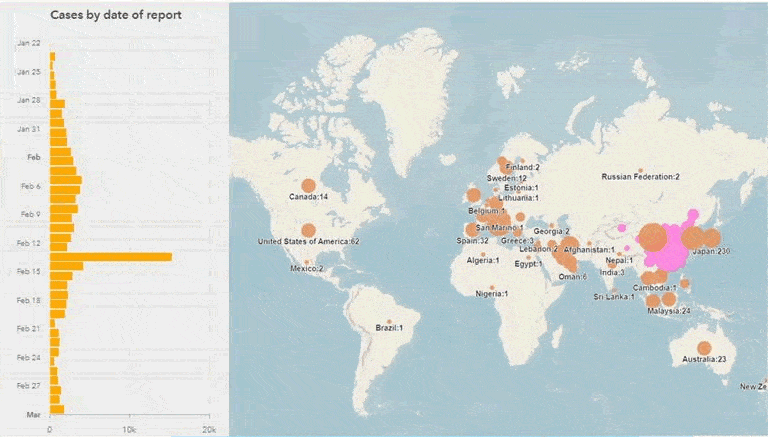
Amino acid composition
Amino acid composition (AAC) is a straightforward but widely used feature descriptor for sequence analysis and model construction. For a total of 20 amino acid types, the AAC descriptor calculates the frequency of each and every type of amino acid. For example, in the event the amino acid type i occurs ni times in the protein sequence, then the frequency of i is denoted by f(i) = ni/L, where L is the protein length. For a given strain, we yielded a 20-dimensional feature vector by computing the frequencies of 20 different amino acids.
Parallel correlation-based pseudo-amino-acid composition
Parallel correlation-based pseudo-amino-acid composition (PC-PseAAC) measures the parallel correlation between any two amino acids in the protein sequence [13]. For a given strain P, the PC-PseAAC feature vector is represented by:
where u is definitely an integer; fvu (1 ≤ u ≤ 20) represents the normalized appearance frequency from the 20 amino acids in the spike protein of P; λ represents the highest tier with the correlation along P; and θj (j = 1, 2, ..., λ) is the correlation function that measures the j-tier sequence-order correlation between every one of the j-th most contiguous residues along P. θj is calculated while using following formula:
where Hm (Pi) (m = 1,2,3,4,5) represents the polarity, secondary structure, molecular volume, codon diversity, and electrostatic charge corresponding to the i-th amino acid Pi within the protein sequence P, respectively [14]. If I + j > L, then I + j equals I + j - L.
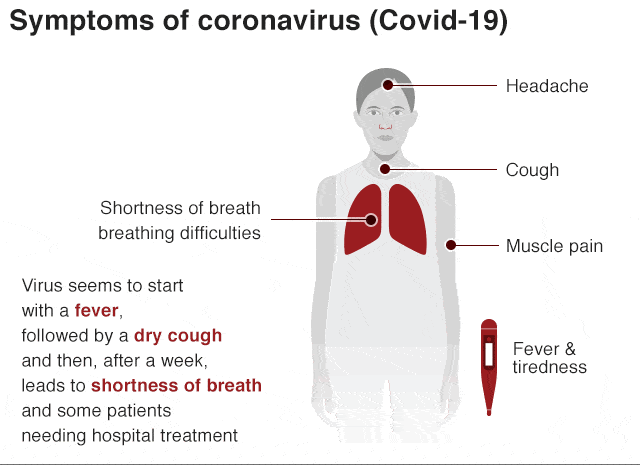
G-gap dipeptide composition
The G-gap dipeptide composition (GGAP) achieves the dipeptide composition as well as local order information from a two interval residues from the spike sequence. It is formulated as
where fvgi may be the occurrence frequency from the i-th (i = 1,2, ...,400) G-gap dipeptide, which is computed as
fvgi=Ogi∑400i=1Ogi
where Ogi represents the occurrence number with the i-th G-gap dipeptide inside spike protein. The dimension of the GGAP feature vector is 20 × 20 = 400.
Machine learning
The framework to the overall prediction is shown in Fig. 1. Two main steps are included: feature representation and machine learning. First, feature representations from three feature descriptors are achieved while using algorithm as described above. Second, the random forest (RF) strategy is used to coach and test the prediction models.
Schematic framework of machine learning. First, feature representations from three feature descriptors are obtained. Second, the RF way is used to teach and test the dataset and make predictions for cross-species transmission of coronavirus. NGDC: National Genomics Data Center; AAC: Amino acid composition; PC-PseAAC: Parallel correlation-based pseudo-amino-acid composition; GGAP: G-gap dipeptide composition; RF: Random forest
As robust and well performance in the field of machine learning, the RF may be widely employed to model biological data. In this research, the RF algorithm is employed to construct models to make predictions for your cross-species transmission of coronavirus. The RF behaves such as an ensemble algorithm and proposes a couple of decision trees, which are grown by the subset of features. The RF repeats the computing process more often than not then is really a final prediction on each sample. The final prediction can merely function as mean of each prediction with bootstrapping algorithm. In this study, the RF algorithm inside R environment was utilized [15]. All the experiments inside study were conducted under R 3.5.0 with default parameters (tree number = 500). To reduce the bias of unbalanced sample number, the positive samples were increased fourfold by the direct duplication of these protein sequences. The 10-fold cross validation method was adopted to judge the predictive performance. Platt scaling was employed to transform the output of the RF model right into a probability over two classes and evaluated chlamydia likelihood of coronaviruses.
Performance evaluation metrics
Four commonly used metrics for model performance evaluation, that is certainly, sensitivity (SN), specificity (SP), accuracy (ACC) and Matthews correlation coefficient (MCC), were chosen inside study. The details are listed as follows:
⎧⎩⎨⎪⎪⎪⎪⎪⎪⎪⎪⎪⎪⎪⎪⎪⎪SN=TPTP+FN×100%SP=TNTN+FP×100%ACC=TP+TNTP+TN+FP+FN×100%MCC=TP×TN+FP×FN(TP+FN) (TP+FP) (TN+FN) (TN+FP)√
where TP indicates true positive, which will be the quantity of correctly predicted true strains with all the phenotype of cross-species transmission; TN represents true negative, which could be the variety of correctly predicted true strains with no phenotype of cross-species transmission; FP represents false positive, which may be the number of strains devoid of the phenotype of cross-species transmission predicted to be strains with the phenotype of cross-species transmission; and FN represents false negative, which could be the quantity of strains using the phenotype of cross-species transmission predicted to be strains devoid of the phenotype of cross-species transmission. The SE and SP metrics look at the predictive ability of the model for bad and the good cases, respectively. The other two measures, ACC and MCC, are used to evaluate the efficiency in the model. Regarding each of the metrics above, the larger their scores, better performance of the model have.
In this research, we also used the receiver operating characteristic curve (ROC) to evaluate the effectiveness of a binary classifier system [16]. It is generated by plotting the true positive rate (TPR) against the false positive rate (FPR) under different classification thresholds. TPR can also be called sensitivity, as described inside above section, whereas FPR could be calculated as specificity.
Results
Screening in the optimal feature
As described in the section Feature encoding algorithms, we used three feature encoding algorithms from multiple perspectives, which is, compositional information and position-related information, as well as physicochemical properties. A total of 41 features were used to practice the prediction models as shown in Table 1. The performances from the protein features were different and the prediction results for your features using the best performance for each and every type are shown in Table 2. As shown in Table 2 and Fig. 2a, the predictive model achieved the utmost ACC of 98.18% coupled with all the MCC of 0.9638 when the feature GGAP (g = 3) was selected. The performance varied from 96.15 to 98.18% for ACC and from 0.9243 to 0.9638 for MCC. This indicated that the feature GGAP with parameter 3 had the optimal representation power to distinguish coronaviruses with assorted phenotypes of cross-species transmission. For the receiver ROC shown in Fig. 2b, the feature GGAP (g = 3) also performed better than another features (PC-PseAAC or AAC). The optimal GGAP feature representation could possibly be explored to observe the evolutionary dynamics of coronavirus.
Predictive performance of feature representations. a Ten-fold cross-validation results. b Receiver operating characteristic curves generated by plotting the actual positive rate (TPR) from the false positive rate (FPR) under different classification thresholds. ACC: Accuracy; SN: Sensitivity; SP: Specificity; MCC: Matthews correlation coefficient; AAC: Amino acid composition; GGAP: G-gap dipeptide composition; PC-PseAAC: Parallel correlation-based pseudo-amino-acid composition
Patterns of human coronavirus
As shown in Table 2 and Fig. 2, the GGAP (g = 3) had the top performance and is also proposed to evaluate the evolutionary dynamics of coronavirus. The features of the 507 human samples in our dataset were chosen showing the patterns with the multidimensional scaling method. Seven clusters for 229E (α-CoV), NL63 (α-CoV), OC43 (β-CoV), HKU1 (β-CoV), MERS-CoV (β-CoV), SARS-CoV (β-CoV), and SARS-CoV-2 (β-CoV) were formed obviously (Fig. 3). The clusters for 229E and NL63 were closed and located within the upper right with the figure. The cluster for SARS-CoV-2 was not far from that for SARS-CoV, this means that both viruses have similar human receptor (angiotensin converting enzyme II, ACE2). The two clusters for MERS and OC43 were far from SARS-CoV and SARS-CoV-2.
Patterns of human coronavirus clustered with all the multidimensional scaling method. The x and y coordinates denote the very first primary factor and second key, respectively. SARS-CoV-2 is indicated from
Evolutionary dynamics of SARS-CoV and SARS-CoV-2
The optimal GGAP feature performed well in terms of predicting infection risk and was used to explore the dynamic of evolution inside a simple, fast and massive manner. Based on the GGAP (g = 3) feature, we computed the Euclidean distance of SARS-CoV-2 and SARS-CoV off their coronaviruses inside dataset to explore the evolution dynamic, separately. As shown in Fig. 4a, the space curve between SARS-CoV-2 as well as other coronaviruses had two gaps. The ‘big’ gap with values from 0 to 0.02 suggests that the SARS-CoV-2 have no close relation to isolated coronaviruses. As shown in Fig. 4b, the distance curve between SARS-CoV along with other coronaviruses also stood a gap worthwhile 0.03, which is comparable to that regarding SARS-CoV-2. The two gaps at 0.03 suggest that coronaviruses near SARS-CoV-2 s or SARS-CoVs form a different group. We further checked the coronaviruses near SARS-CoV-2 and SARS-CoV (< 0.03) and found these close relatives were a similar.
The results were similar to those from the MDS method and confirmed that SARS-CoV-2 s and SARS-CoVs have the same origin. Moreover, the top gap at 0.02 shows that the original source of SARS-CoV-2 s just isn't clear and further surveillance in the field must be made continuously. The smooth curve for SARS-CoVs implies that its close relatives still exist naturally and public health is challenged as usual.
To read more about Coronavirus Essay Topics take a look at https://www.nhs.uk/conditions/coronavirus-covid-19/